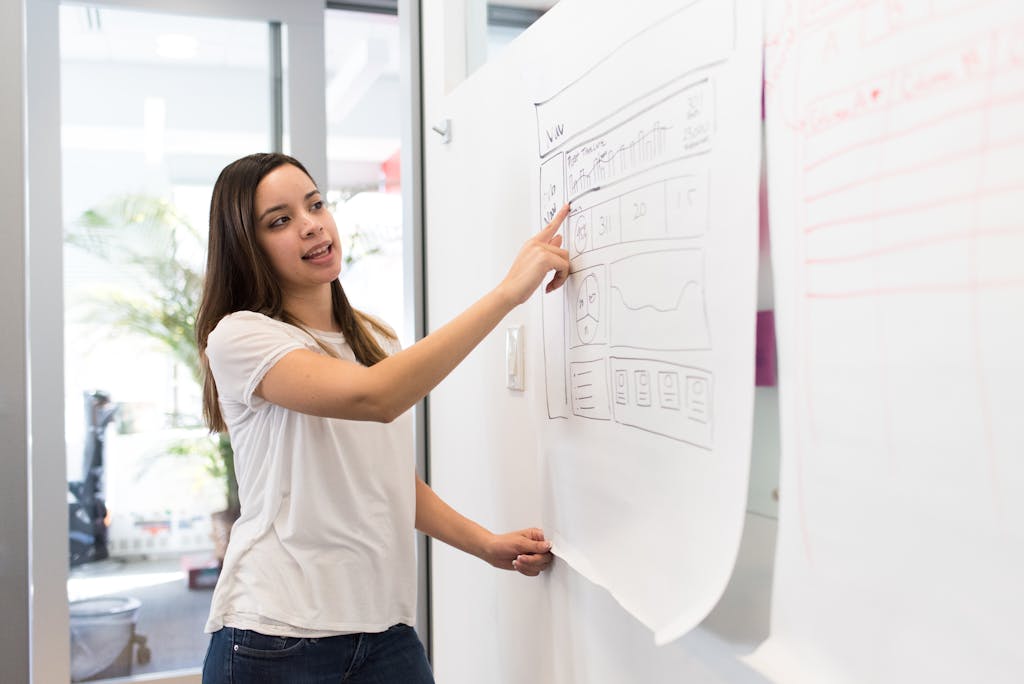
Forecasting the Future of Market Research
Filed Under: Best Practices, Market Research, Tools & Techniques
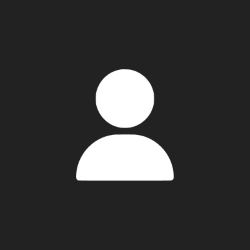
Walt Dickie
One of the big events of my formative years happened around 1967 at MIT when the guys in the Earth Science Department announced that they could “predict” what the weather was like in Cambridge an hour earlier.
What actually happened was that a meteorological model had been developed that forecast the weather pretty accurately 24 hours in advance. The problem was that the model took a little over a day to execute on the mainframe, so by the time it issued a forecast it was “predicting” the weather we had just experienced. It was a geeky story, and everyone I knew thought it was pretty funny. A computer model had been developed that was as good at forecasting the weather as looking out the window.
But we all knew that it was a really big deal. With improvements to the algorithm and some advances in the hardware, it would only be a matter of time before the model would run in an hour, then a minute, and it would soon be capable of forecasting the weather not 24 hours in advance but 48 hours, or a week, a month … who could guess?
I’ve watched the weather my whole life because I’ve always been addicted to outdoor sports – I ride a bike almost daily outside of the three- or four-month period when the Chicago winter drives all but the insane indoors. I’ve been a skier since childhood, as has my wife, and we’ve passed that mania on to our kids, so that gives us a reason to follow the winter weather. And I was bitten by sailing when growing up on a New England lake, which now takes out on Lake Michigan and, again, has me pouring over the weather sites.
Historically, there have only been a few ways to forecast future weather. Until very recently forecasters struggled to consistently beat the algorithm my mom used when I was a kid: “Tomorrow will be pretty much like today.” If you think about your local weather, you’ll probably see what we see here in Chicago – the weather mostly doesn’t change much from one day to the next, until it flips a switch and changes a lot. Fronts move through every few days – but in between, tomorrow is much like today. You might be right with that algorithm as often as 3 days out of 4, or even 4 out of 5. It was a struggle to develop a meteorological system better than that.
As recently as the early 20th century, “forecasters would chart the current set of observations, then look through a library of past maps to find the one that most resembled the new chart. Once you had found a reasonably similar map, you looked at how the past situation had evolved and based your forecast on that.”[1] When the technology appeared giving forecasters a reasonably complete picture of today’s weather “upstream” from their location, they were able to adopt a variation on this technique and base tomorrow’s Chicago forecast on what was happening on the Great Plains today, rather than relying on an old map.
And then came the modelers’ breakthrough – the algorithm that forecast the weather an hour ago – and soon it was possible to base forecasts on scientific principles and mathematical calculation.
So, vastly simplified, the evolution of weather forecasting was this: predict that tomorrow will be like today, predict that tomorrow will be like it is today somewhere else, and, finally, calculate tomorrow’s weather by mathematically extrapolating the underlying physics of today into the future.
I’ve been thinking about this progression recently because C+R, like most MR firms these days, is spending an increasing amount of time trying to predict the future. The industry is changing; the economy is changing; maybe the entire global financial system is changing; technology is certainly changing. How do we navigate? How do we make business decisions for the future without some way of forecasting the future?
Everyone here is thinking about these issues, but there are three of us who are particularly involved because of our job responsibilities. And we’ve discovered that each of us has a personal method for forecasting.
Partner Number One is heavily, almost exclusively involved in sales, and has constant contact with clients and potential clients who are trying to articulate their research needs. Partner Number Two monitors the new products and services that our competitors and the industry as a whole are introducing, paying particular attention to successful leading-edge competitors. And Partner Number Three monitors emerging technology and social trends and tries to infer their likely impacts.
And guess what? Partner Number One says that, as near as can be told, tomorrow is going to be a lot like today. Although there is a lot of discussion about big changes online, at conferences, in speeches, and in trade publications, the projects that clients need today and expect to need in the immediate future are much the same as they’ve been in the recent past. Timelines are shorter and budgets may be tighter, but tomorrow’s weather looks like today’s.
Partner Number Two sees a lot of new product and service activity going on, and notices what seem to be some really amazing storms and lightning bolts as new firms with new offerings post double-digit growth rates year upon year while others seem to explode only to fizzle. Some amazing things are announced and then never heard from again. It seems like we can look around us on the map, but that it’s really hard to know which direction is “upstream” from where we’re located. It’s hard to tell if the weather being experienced elsewhere on the industry map will travel toward us or away from us.
Partner Number Three sometimes seems to detect solid trends. There really seems to be some clear trends in technology – both the technology that our client businesses are adopting and the technology that consumers are using. Some trends in consumer communication technology seem especially clear, and if data collection will play any role in our future then we can base that part of our forecast model on them. But other areas, particularly the “information ecology” of businesses seem roiled up and hard to read. We have some pieces of a prediction model, but our algorithm for forecasting still needs a lot of work. It’s not clear that we’re anywhere near the point I witnessed back in college when the model first beat my mom’s forecasting approach.
I find myself torn between algorithms. Like the weather, many businesses have had one day follow another with little material change for long periods – until change overtakes them like a summer squall. Been to a bookstore lately? Just because an innovation lit up the landscape somewhere doesn’t necessarily mean that the same thing would happen elsewhere, or that the market would support two, three, or a dozen similar offerings. Maybe a competitor’s success is due to local conditions – like updrafts that spawn tornadoes on the Plains but almost never come east to Chicago. And although the trends seem to point toward weather systems coming in soon, the forecast model isn’t any better at this point than looking out the window and expecting more of the same.
So we try a little of each forecaster’s method, experimenting with the trends and borrowing from competitor’s successes, while finding today’s weather still largely unchanged from yesterday’s.
[1] Paul N. Edwards, A Vast Machine, MIT Press, 2010, p. 89. This book, about climate modeling, is one of the 10 finest books I’ve read in the last decade. It is not only a marvelous discussion of climate; it is the best general book about modeling and data management I can imagine.
explore featured
Case studies
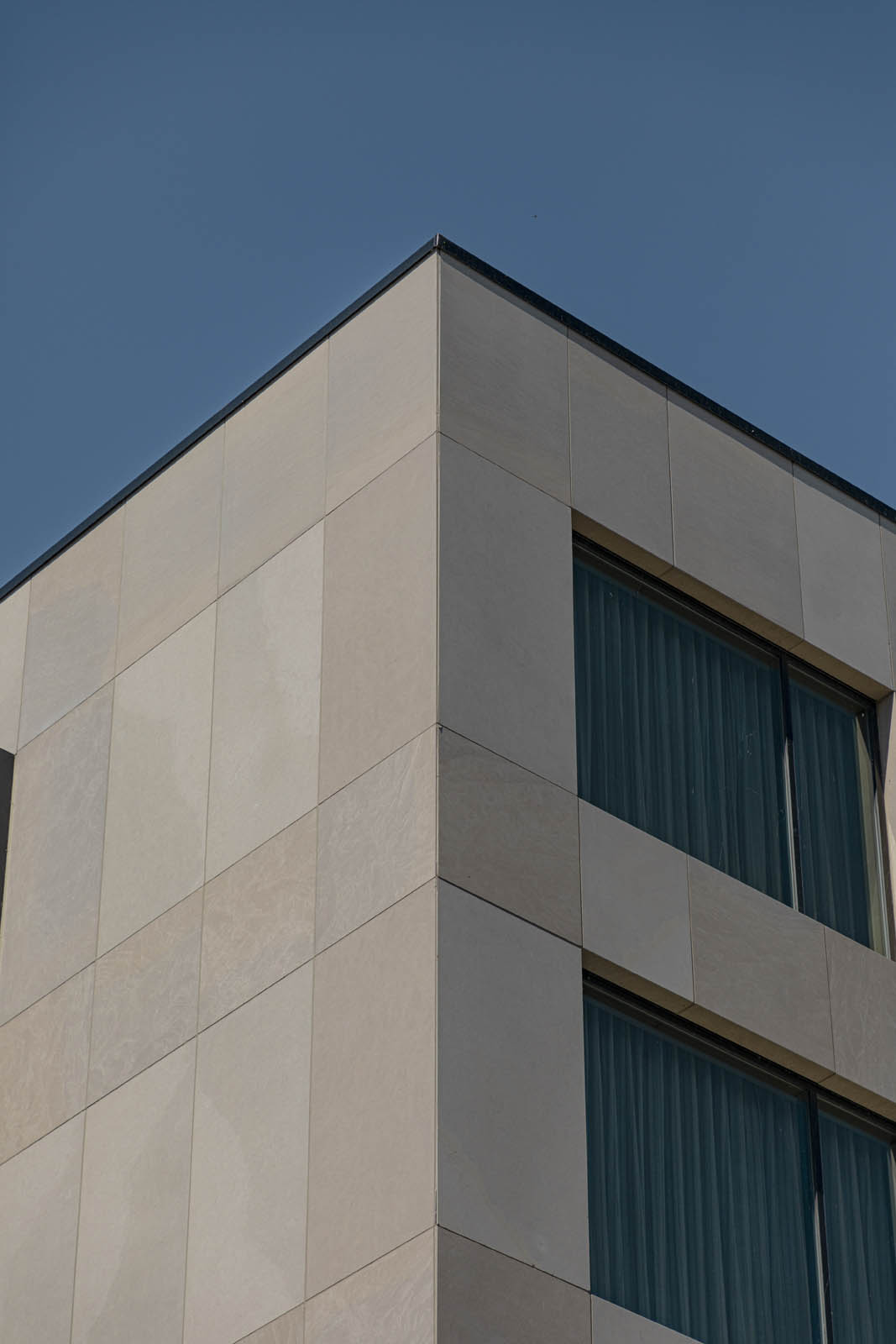